Back in the good ol’ days, before we talked about content curation as a next-generation web service, we lived in a curated web. Yes that’s right. Long before there were social networks, even before there were mainstream search engines, people found content online by navigating catalogs of carefully curated content, collections of hand-picked links, or hierarchies of meticulously organised pages. Back in the day, Yahoo! was the king of the curated web, providing millions of early web adopters with access to a carefully curated hierarchical catalog of pages and links. The catalog still survives today even though, by and large, we web users search for, rather than navigate to, the content we seek, but nonetheless in the pre-dawn of the modern web people located content by navigating through Yahoo!’s comprehensive catalog. Of course, then along came Google. And the rest, as they say, is history.
Whitepaper: Towards the Reputation Web
Since the dawn of the Internet we have used ratings as a proxy for reputation to guide our activities; buyer and seller ratings, for example, have been crucial to the success of eBay and similar online markets. But as the web has become more social, shifting the emphasis from pages to people, we will see reputation playing an ever more crucial and pervasive role to mediate and qualify our interactions.
In this white paper we consider some of the implications of the ability to measure and use reputation, particularly as a recommendation signal in the world of information discovery and collaborative web search.
Reputation Inc. – The Value of Social Reputation on the Recommendation Web
Way back in the pre-Google dawn of the 1990’s Internet there was a much heralded approach to web search by a company called DirectHit. The message was simple: paying attention to the words in a document (and query) was not enough to do a good search job, we need to pay attention to the results people select.
To be fair, the first part of this idea – that the words or terms in a query and document were not enough – was accepted by then; at the time a couple of grad students at Stanford were doing some interesting things with links as a result ranking signal for the same reason. But where Boston-based DirectHit differed was it’s emphasis on engagement signals. For instance. the Direct Hit search engine harnessed the searching activity of millions of anonymous web searchers to rank websites based on often searchers selected a page, how long searchers spent viewing it, and where the page was ranked in the original search results list. Ultimately, Direct Hit’s so-called Popularity Engine ranked search results based on a formula that combined a variety of engagement signals to evaluate the page’s popularity. At the time the idea was fascinating and potentially powerful; so much so that Direct Hit was acquired by Ask Jeeves for more than $500m in stock.
Whitepaper – Mobile, Social Search: A Case Study
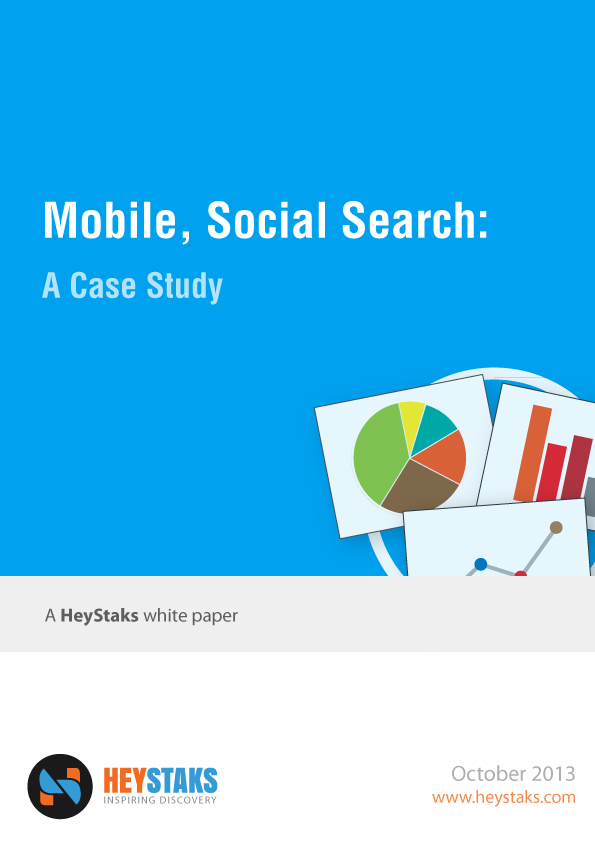
By Prof. Barry Smyth, HeyStaks’ Chief Scientist
We are living in a discovery economy where access to the right information at the right time can make the difference between success and failure. The ability to use modern information discovery tools such as search engines, social media, and related services is an important skill for us all to master, particularly on mobile devices where new tradeoffs exist when it comes to searching for and finding information online. In this paper we describe one such solution in the form of collaborative search, which combines conventional term-based search with a more social approach to information discovery that is particularly well-adapted to the constraints of mobile devices.
Public vs Private Parts: Personas & Sharing on the Recommendation Web
The Internet is an archive of our lives. Every event captured, every photo rendered, and every conversation indexed. And, if it can be stored it can be found. Maybe not today but someday, by a friend perhaps, or a future employer, whether we want it to be found or not. This is both liberating and terrifying. But is it creating an incentive for people to hide their true personalities? Are we curating carefully crafted personas online that only disguise our genuine personalities? If so then, what we share may not be what we click and this has some important implications for the future of personalization, sharing, and recommendation on the web.
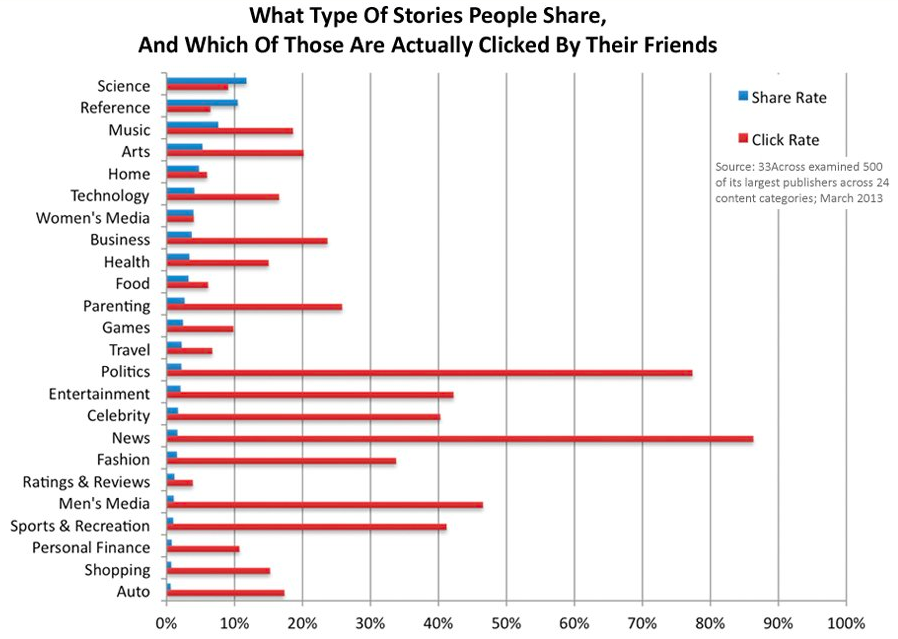
Figure 1. An analysis of what people share versus what is clicked, by 33Across, and based on 450 large publishers and 24 content categories.
Whitepaper – Social Search and Search Analytics in the Discovery Economy: An Enterprise Perspective
Knowledge workers continue to struggle when it comes to finding the right information at the right time, leading to high search failure and abandonment rates – 50% of queries lead to failed searches and 44% of knowledge-workers fail to find what they are looking for – a significant cost to enterprise in terms of lost productivity and missed opportunities. One practical solution is for a more collaborative approach to search, which harnesses the past search patterns of experts within an organisation, and works in tandem with conventional search services to provide more relevant and useful results. By harnessing the power of collaborative search, HeyStaks can improve search effectiveness within the enterprise by up to 50% and by fostering improved collaboration HeyStaks will improve engagement, knowledge sharing, and innovation right across an enterprise.
The Evolution of Web Search:From Real-Time Discovery to Collaborative Web Search
Certainly the world of the Web has changed dramatically since 2000, and search engine technology has evolved through a variety of phases. For example, in the pre-Google dawn (Search 1.0), search engines were guided primarily by the words in a page, their location and how they matched the query terms. Google’s great innovation was to demonstrate how search quality could be greatly enhanced by harnessing a new relevance signal: the links between pages. Google’s link analysis technology (PageRank) interpreted links to a page as votes and PageRank was a clever way of counting such votes to effectively compute an authority score for each page, which could then be used during result ranking.As an aside, back in the late 1990’s one of Google’s fellow innovators was a company called Direct Hit, which also argued for the need for new relevance signals. But in the case of Direct Hit the focus was on paying attention to how often users selected a page for a given query, something we will return to later. In the end Google’s PageRank was the right search technology at the right time and the rest, as they say, is history. And so Search 2.0 was primarily driven by relevance signals (links, click-thrus) that originated beyond the content of a page. More recently we have seen further innovation in the direction of vertical search (arguably Search 3.0) for topics such as images, travel, products etc. and the blending of different types of result within a universal search interface (see for example, Google’s Universal Search.