The Internet is an archive of our lives. Every event captured, every photo rendered, and every conversation indexed. And, if it can be stored it can be found. Maybe not today but someday, by a friend perhaps, or a future employer, whether we want it to be found or not. This is both liberating and terrifying. But is it creating an incentive for people to hide their true personalities? Are we curating carefully crafted personas online that only disguise our genuine personalities? If so then, what we share may not be what we click and this has some important implications for the future of personalization, sharing, and recommendation on the web.
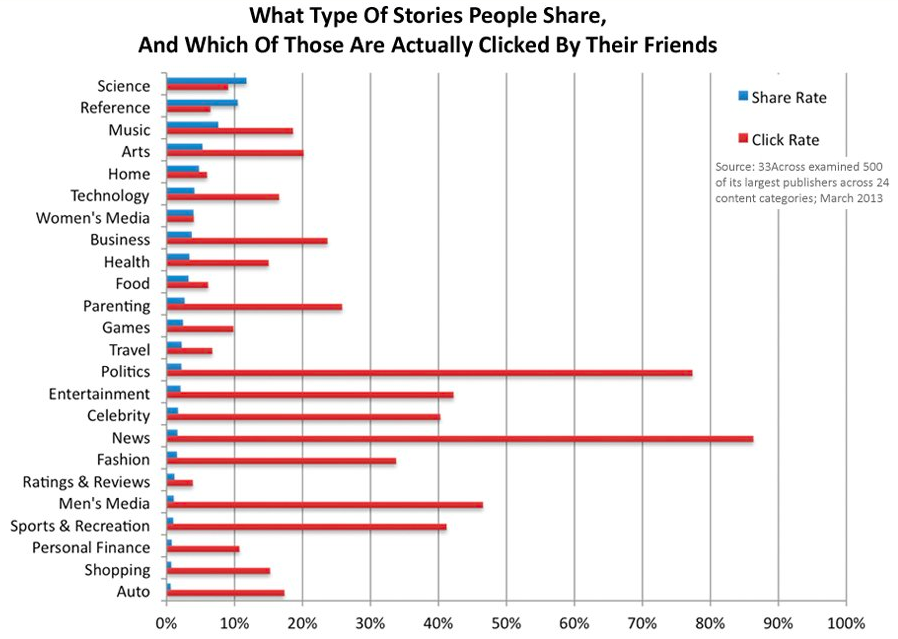
Figure 1. An analysis of what people share versus what is clicked, by 33Across, and based on 450 large publishers and 24 content categories.
Share ≠ Click
Figure 1 illustrates the sharing and click-through data collected by 33Across during one recent analysis of what we share and click online. It reveals several counterintuitive findings about what motivates people to share content with others. In particular, people aren’t necessarily sharing what their friends or followers want to read. For example, people shared science articles at a higher rate (12%) than articles on any other subject, but these articles were clicked only 9% of the time, compared to an average click rate of 24% across all other subjects.
Common sense suggests that content that is frequently shared should also be frequently read. However, the 33Across data indicates otherwise. Is this a sign that our sharing habits are at odds with our genuine interests? Do we share science related content to make ourselves feel smart, while surreptitiously clicking on celebrity gossip because nobody will know? Why do we only share shopping related content less that 1% of the time when it attracts 15% of the clicks? Is what we share driven more by our ego and our desire to be identified with certain topics rather than our genuine interests or those of others?
Implications for Recommender Systems
This dichotomy between what we share and what we click may have profound implications when it comes to building recommender systems and other forms of personalized online services. To be successful, recommender systems must develop an authentic picture of our preferences and interests. Only then can they generate truly relevant recommendations. In the past our profiles have been mined from our online behaviours, often using implicit indicators of interest (e.g. what we click, read, watch, purchase online) which are then converted into ratings. These ratings are used by collaborative filtering algorithms to generate lists of recommendations from the past likes of users whose profiles match our own.Typically we don’t know these similar users – algorithms determine similarity by comparing ratings histories – they just happen to have similar profiles with ratings that are closely correlated with our own.
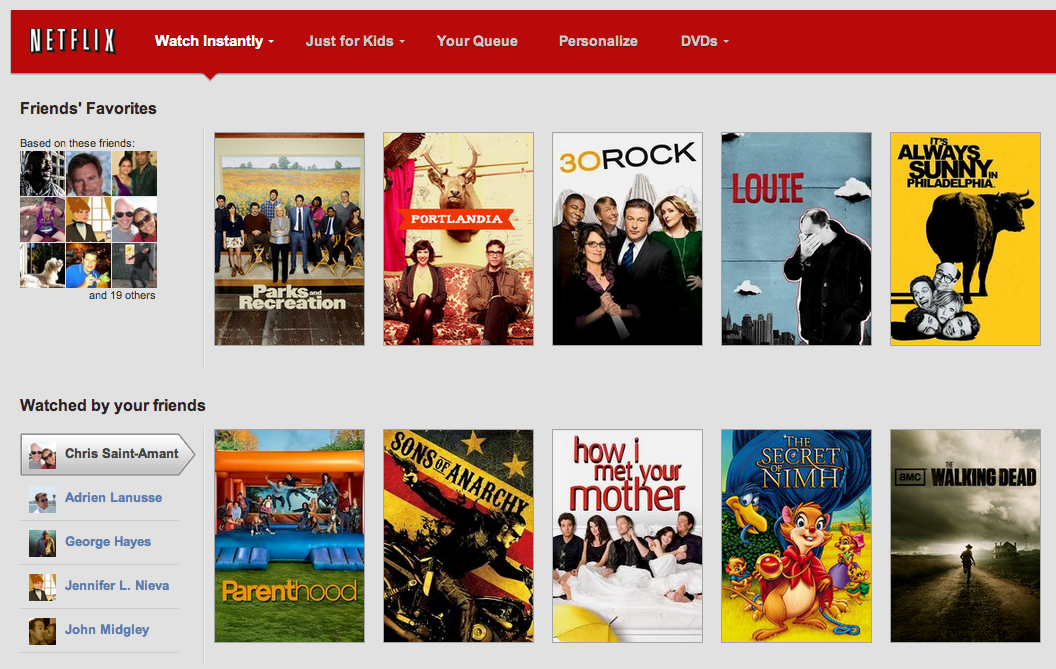
Netflix now prioritizes social recommendations alongside algorithmic suggestions.
More recently however recommender systems have been built based on new sources of profile and similarity data. In particular, the availability of mature social graphs (a la Facebook, Twitter etc) has led to a new breed of social recommender systems (as distinguished from the algorithmic recommender systems above), which now draw their suggestions from a user’s friends, friends of friends, and what they share. For example, the recommender system powerhouse that is Netflix, now prioritises such social recommendations, organising it’s interface around the recommendation from our online friends.
The intuition here is clear: basing recommendations on actual friends must surely trump suggestions derived from algorithmic matches? That may be the case. Perhaps it helps users to better evaluate recommendations knowing, as they do, something about their friends true tastes and preferences. It may work well in certain domains, but ultimately caution is needed. If these social recommender systems use what we share as the raw material of our profiles then will they produce an authentic picture of who we are? Or do our social network personas reflect a mere shadow of our true interests and preferences: just those things we want to be known for rather than those things we are actually interested in? If this is the case then the recommendations that result may be as bland and brittle as the personas we often project in public; see also “The Masks Men Wear” and “The False Self“.
Authentic Recommendation Signals
At the very least all of this highlights the need for additional signals with which to guide recommendation relevance. In HeyStaks we give a voice to search queries and result selections, because what we search for and click on is likely to be a more authentic reflection of our genuine interests and preferences. But we can go further too. As we will discuss in a future post, by understanding an individual’s authentic interests and by monitoring how others respond to these interests we can start to model their reputation online and across multiple communities. Such reputation scores can provide recommender systems with new insights by signalling not just what an individual is interested in but also their influence over others, which in turn can lead to more focused and insightful suggestions and recommendations. Stay tuned for Part 2 …