Search Engines and Search Behaviour are Changing. What was once the focus of static individual search is now becoming dynamic social search.
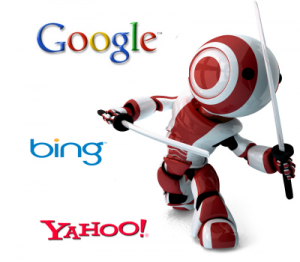
Since the inception of web search (1990 to the present day – 23 years), the ways in which search engines work, and how people use them, have changed in tandem. Smarter algorithms, improved technology and greater user demand for higher quality results merit search engines becoming smarter in order to survive. This timeline shows an interesting view of how many, and what type of, search engines have become active (and in many cases inactive) over the past 20+ years.
The first search engines:
Not only have the search engines changed, user search behaviours and expectations have also changed. Initially, web users were technical and had little need to search – they primarily knew where the content they needed lived. Search engines at this time merely looked at URL’s and not page content so relevance/search quality was a major issue. As all relevant search terms could not be placed in the URL, search quality and satisfaction placed much more emphasis on the user entering terms that could be matched against the URL. Searches were mainly informational – search was only exposing query term(s) that exist on some website. Search engines evolved to also index page content – this meant that end users could now query terms that matched content of pages. Searches were still based on the informational needs mentioned above. This also led to bias, spam and lower relevance as it was very easy for site authors to overload search terms.
Research from this era of search showed that users had basic search demands. Analysis of user searches in Searching The Web: The Public and Their Queries showed:
- Average query length was 2.4 search terms.
- 50% of users entered a single query while a < 33% of users entered 3+ unique queries.
- 50% of users looked at less than 2 pages of results
- Less than 5% of users used advanced search features (e.g. boolean searches).
- The top four most frequently used terms were “<> ” (empty search), and, of, and sex.
Looking ‘outside the page’:
A (generational) shift now occurred in the search space. Companies like Google & Direct Hit started placing more emphasis on “external-to-page” content such as click through data, anchor text and link analysis. While this did not directly affect the way in which users search, users adapted to deal with the improving quality of search results. Ironically, they did this by changing their search language to discover results which were no longer present due to these ‘next generation’ algorithm changes. An example of a ‘first generational’ search query for “restaurant london” now evolved to a more targeted ‘second generation’ query like ‘restaurant london waterloo chinese’. Users refined and rephrased their search queries much more to get the results they wanted. They also started to use more advanced techniques such as boolean searches combined with result filtering using content metadata such as date, language and file type. Research from this period of search also showed that query term count had increased dramatically from previous research. Demonstrating that there is much more demand for increased query term length, Google revealed a video from their search quality team discussing search improvements on queries containing up to 13 terms.
Time to become more social:
The latest shift in search technologies has focused on the social innovations in online technologies to help counteract some limitations. As described by Stefan Weiz, Director of Search at Bing:
“search engines can really only offer query results if you’ve perfectly described for the engine what it is you don’t know.”
Search engines are at a stage where ‘standard’ techniques (link analysis, information retrieval and semantic analysis) is proving to be the blocker for improved results. Users queries are shifting towards much more natural query styles such as “question search”.
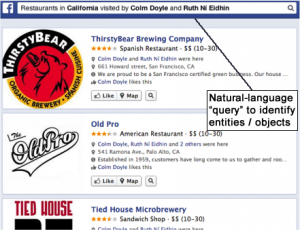
Combining this with the fact that we now have a long tail query problem and real time search is increasing the size of result sets, search engine algorithms now aim to make use of real time personal interactions ( search context signals) and social interactions to improve relevance. While traditional search engines may be limited by ‘perfect search queries’ and ‘what you don’t know’, newer search engines like HeyStaks place much more emphasis on user feedback and community information to discover what a user wants at the time they search. This community information (based on ‘like minded’ users with similar interests) can fill in the ‘query blanks’ – those vital potentially missing pieces of information that can change a failed or low quality search to the ‘perfect’ result list for that user.
And that, at the end of the day, higher search quality is what every search engine should aspire to.